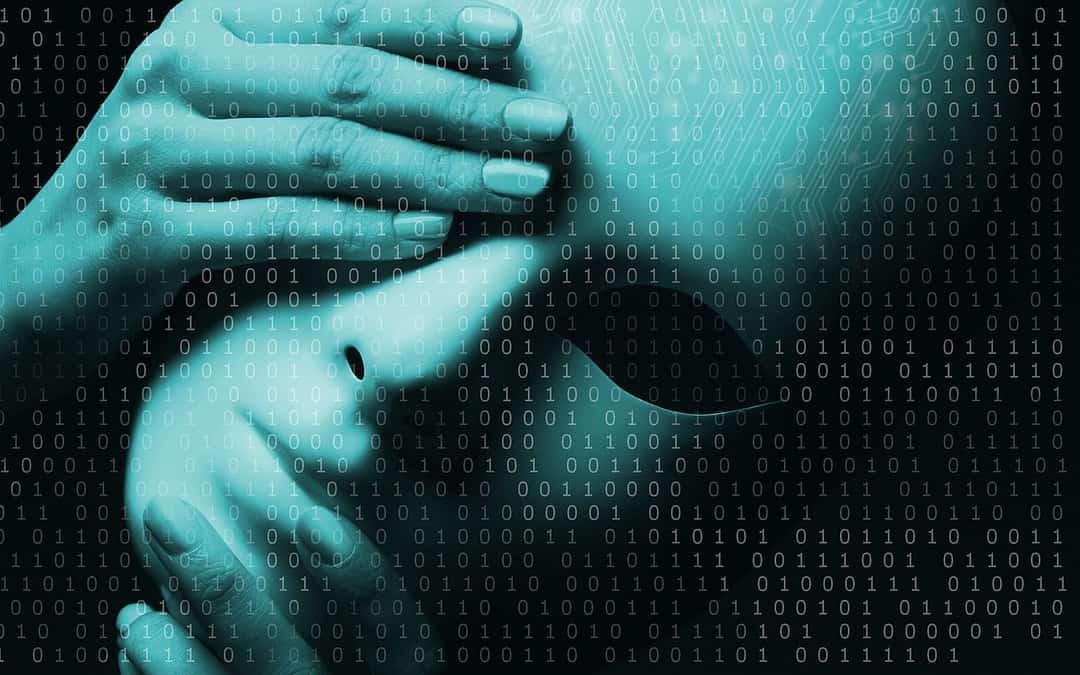
Artificial Intelligence V Hinton The concerns of the “father” of Artificial Intelligence
Geoffrey Hinton, a true legend in the field, now believes that the technology he helped develop could lead us to the end of civilization in a matter of years.
The topic of Artificial Intelligence, with its vast reach and the little we actually know – in many cases, we are still in an intuitive stage – has led to an authentic flood of studies, opinions, controversies, and heated debates that are practically occurring daily.
Our Laboratory believes that one of the best services we can provide to all those people and organizations following our work is to offer a selected series of those opinions, positions, and debates, brought practically up to date on the day they occur, to keep genuinely informed those who are attentive to what is happening and to our vision.
By the way, the Laboratory is working on its Artificial Intelligence Microlab and will timely share its conclusions and perceptions, but the urgency of the issue does not allow for too many delays. That is why we are launching a series on Artificial Intelligence today, which we hope will be the catalyst for analysis, reflection, and conclusions on the projection that a topic of this magnitude forces us to address. No one, neither governments, nor international organizations, nor regional bodies, think tanks, and individuals can remain indifferent to its evolution.
As always, we hope that our service can be of use to you.
Geoffrey Hinton is extremely concerned about the future of artificial intelligence:
Outside the field of artificial intelligence research and development, many people may not have heard of Geoffrey Hinton, perhaps the pioneer or one of the most prominent members of the group of pioneers who developed and systematically worked on artificial intelligence. But this author and his thoughts represent a milestone in this entire great discussion we are going through, and it matters to us because his opinion is decisive for decision-making, especially of a public nature, and his opinions, whether positive or negative, cannot be ignored. Indeed, the specialist has recently gone through a highly significant personal and professional event – he just resigned from his job at the giant Google. A decision that has deeper roots than a mere whim or a simple option between yes or no.
Hinton, at 75 years old, has seen many things in his professional career and personal life. He has been both a protagonist and a witness, not only to the development of computing but also to the birth and development of the Internet, building his life upon it. For this reason, and because of his vast scientific career, it is essential to pay attention to his vision in times when the discussion about the topic, its consequences, and its potential regulation is intensifying. We must be careful about the discussion and the argumentative lines being developed: we know we are facing a matter of immense importance.
Introducing Hinton:
He was born in Wimbledon, UK, on December 6, 1947. He holds dual nationality, both from his country of origin and his country of residence, Canada. He was educated at the University of Edinburgh and holds a Ph.D. in Artificial Intelligence. After obtaining his degree, he worked at the University of Sussex – where he faced some funding difficulties – and emigrated to the University of California in San Diego and Carnegie Mellon University.
He was the founding director of the Gatsby Charitable Foundation’s Computational Neuroscience Unit at University College London.
His area of work for a long time has been Deep Learning and Machine Learning. In addition to theoretical computer science, his occupations include being a university professor at the University of Toronto (in the Department of Computer Science) and at Carnegie Mellon University, where he taught until 1987.
He holds the Canada Research Chair in Machine Learning and is currently an advisor for Machine Learning and Brains at the Canadian Institute for Advanced Research. He joined Google in March 2013 when his company, DNNresearch Inc., was acquired.
As noted, he worked at Google until he recently chose to leave, citing the dangers he recognizes in certain new technologies and the insistence of some companies to continue down a path that leads to those dangers, most of which have not been fully measured in their depth. He made this decision at the age of 75, in a society deeply dismissive of its older theorists.
Additionally, he is a member of the American Academy of Arts and Sciences; the American Association for Artificial Intelligence; the European Laboratory for Learning and Intelligent Systems.
Hinton was elected a member of the Royal Society (FRS) in 1998. He was the first recipient of the David E. Rumelhart Prize in 2001.
In 2001, Hinton was awarded an honorary doctorate from the University of Edinburgh. In 2005, he received the IJCAI Research Excellence Award for his career. He was also awarded the Herzberg Gold Medal in Canada for Science and Engineering in 2011. In 2013, Hinton was awarded an honorary doctorate from the University of Sherbrooke, also in Canada.
In 2016, he was elected a foreign member of the National Academy of Engineering “For his contributions to the theory and practice of artificial neural networks and their applications to speech recognition and computer vision.” He also received the IEEE/RSE Wolfson James Clerk Maxwell Award in 2016.
He has won the BBVA Foundation Frontiers of Knowledge Award (2016) in Information and Communication Technologies for his “pioneering and highly influential work” in endowing machines with the ability to learn.
He won the Turing Award in 2018 (considered the Nobel Prize in Computing) together with Yoshua Bengio and Yann LeCun for their work in Deep Learning, particularly for conceptual and engineering advances that have made deep neural networks a critical component of computing.
Currently, Professor Hinton is researching ways to use neural networks for machine learning, memory, perception, and symbol processing. He is the author or co-author of over 200 peer-reviewed publications in these areas. While at Carnegie Mellon University (1982-1987), Hinton was one of the first researchers to demonstrate the use of the generalized backpropagation algorithm to train multilayer neural networks, which has been widely used for practical applications. During the same period, Hinton co-invented Boltzmann machines with David Ackley and Terry Sejnowski. His other contributions to neural network research include distributed representations, time-delay neural networks, expert mixtures, Helmholtz machines, and Product of Experts. In 2007, Hinton co-authored an unsupervised learning paper titled “Unsupervised Learning of Image Transformations.” An accessible introduction to Geoffrey Hinton’s research can be found in his articles in Scientific American in September 1992 and October 1993.
Hinton moved from the U.S. to Canada partly due to his disillusionment with Reagan-era politics and disapproval of military funding for Artificial Intelligence. He believes that political systems will use AI to “terrorize the population.” Hinton has petitioned against lethal autonomous weapons. Regarding the existential risk of AI, Hinton has stated that superintelligence seems to be more than 50 years in the future, but he warns that “there is no good trajectory of less intelligent things controlling things of greater intelligence.” When interviewed in 2015 and asked why he continues his research despite his serious concerns, Hinton stated, “I could give you the usual arguments. But the truth is that the prospect of discovery is too sweet.” Hinton has also stated that “it’s very hard to predict beyond five years” what advancements AI will bring. In fact, the goals he envisioned regarding timelines have been drastically changed by him. Until recently, Hinton’s prediction was that AI would surpass human intelligence in about thirty or fifty years, but the evidence shows that this time has been reduced to between five and twenty years.
“If there is any way to control artificial intelligence, we must figure it out before it’s too late.”
There is a set of reasons why Hinton left his position as Vice President of Engineering at Google and started a campaign to warn that the world must fear the technology.
His current intention is to dedicate himself to warning about what he considers the dark side of Artificial Intelligence (AI), according to what he said in an interview with the prestigious and influential The New York Times. This “crossing” from one side of the bridge to the other is not simply a change of opinion from someone. Hinton is a legend in the field. His work has been decisive in developing some techniques that made possible ChatGPT, automatic translators, or vision systems for autonomous vehicles. He has crossed the bridge because he now firmly believes that the technology he helped develop so much could lead us to the end of civilization within years.
This is not a whimsical conclusion; this scientist’s obsession has always been to study how the brain works to try to replicate those mechanisms in computers. In 1972, he coined the concept of a neural network. The underlying idea was originally to apply mathematics to data analysis so that systems could develop skills. His proposal was not convincing at the time; today, neural networks are the spearhead of research in Artificial Intelligence. Hinton’s breakthrough came in 2012 when he demonstrated the true potential of his research line with a neural network that could analyze thousands of photographs and learn by itself to distinguish certain objects, like flowers, cars, or dogs. He also trained a system to predict the next letters of an unfinished sentence, a precursor to current large language models like ChatGPT.
The dangers we face, both immediate and “existential”:
According to Hinton’s current thinking, we face many dangers. The generation of fake news is already causing great divisions in society. The elimination of certain types of jobs will impact employment. The wealth disparity between the rich and the poor will increase. These are some of the imminent dangers, although there are other existential ones. According to Hinton, “I recently realized that the type of digital intelligence we are developing could be a form of intelligence better than that of biological brains. I always thought that AI or deep learning tried to imitate the brain, although it couldn’t match it: the goal was to improve so that machines became more and more like us. I’ve changed my stance in the last few months. I believe we can develop something much more efficient than the brain because it is digital.” And what this statement covers is what we should consider truly concerning.
He believed that, in any case, we could imitate the brain, but now he believes we can create something more efficient than the brain because it is digital:
With a digital system, we could have many copies of exactly the same model of the world. These copies can work on different hardware. In this way, different copies could analyze different data. And all these copies can instantly know what the others have learned. They do this by sharing their parameters. But that’s not possible with the brain. Our minds have learned to use all their properties individually. If I gave you a detailed map of the neural connections in our brain, it would be useless. But in digital systems, the model is identical. They all use the same set of connections. So when one learns anything, it can communicate it to the others. And that’s why ChatGPT can know thousands of times more than any person: because it can see thousands of times more data than anyone. That’s what scares him. Maybe this form of intelligence is better than ours.
He comes to this conclusion after trying to figure out how a brain could implement the same learning procedures used in digital intelligences like those behind ChatGPT-4. Based on what we know so far about how the human brain works, our learning process is probably less efficient than that of computers.
Can Artificial Intelligence be “truly intelligent” if it doesn’t understand what words mean or have intuition?
Deep learning, when compared to Symbolic AI (the dominant paradigm in the field until the rise of neural networks, which tried to make machines learn words and numbers), is a model of intuition. If we take symbolic logic as a reference, if we believe that’s how reasoning works, we can’t answer the question that needs to be asked. But if we have a computational model of intuition, the answer is obvious. So here’s the question: we know there are male and female cats. But let’s suppose I say you must choose between two ridiculous possibilities: all cats are male and all dogs are female, or all cats are female and all dogs are male. In our Western culture, we’re quite clear that it makes more sense for cats to be female because they are smaller, smarter, and surrounded by certain stereotypes, and that dogs are male because they are bigger, less intelligent, louder, etc. I repeat, it makes no sense, but forced to choose, I think most would say the same. Why? In our minds, we represent cats and dogs, men and women, with large patterns of neuronal activity based on what we’ve learned. And we associate the representations that most resemble each other. That’s intuitive reasoning, not logical. This is how deep learning works.
Is it possible for Artificial Intelligence to have its own purposes or objectives?
Here is a key question, perhaps the greatest danger surrounding this technology. Our brains are the product of evolution and have a series of built-in goals, such as not hurting the body, hence the notion of damage; eating enough, hence hunger; and making as many copies of ourselves as possible, hence sexual desire. Synthetic intelligences, on the other hand, have not evolved: we have built them. Therefore, they do not necessarily come with innate objectives. So the big question is, can we ensure that they have goals that benefit us? This is the so-called alignment problem. And we have several reasons to be very concerned. The first is that there will always be those who want to create soldier robots. This can be achieved more efficiently by giving the machine the ability to generate its own set of goals. In that case, if the machine is intelligent, it won’t take long to realize that it achieves its goals better by becoming more powerful. So one of the key points is to put as much effort into developing this technology as into ensuring it is safe.
The paths to develop now:
It is necessary to draw people’s attention to this existential problem posed by Artificial Intelligence. I wish there were a clear solution, as in the case of the climate emergency: we need to stop burning carbon, even though there are many interests preventing it. But no equivalent problem to Artificial Intelligence is known. So the best we can do right now is to put as much effort into developing this technology as into ensuring it is safe. And that’s not happening currently. But how do you achieve this in a capitalist system?
Google developed chatbots like LaMDA, which were very good, and deliberately decided not to release them to the public because they were concerned about their consequences. And so it was while Google led this technology. When Microsoft decided to put an intelligent chatbot in its Bing search engine, Google had to respond because they operate in a competitive system. Google acted responsibly. Hinton doesn’t want people to think he left the company to criticize it. He left Google to warn about the dangers without having to worry about the impact it might have on their business.
The general situation of the scientific community and manifest naivety:
Hinton’s conclusions are that we have entered completely unknown territory. We are capable of building machines stronger than us, but we still have control. But what would happen if we develop machines smarter than us? We have no experience dealing with these things. We really need to think a lot about this. And it is not enough to say that we won’t worry. Many of the smartest people we know who are working on these issues are seriously concerned.
It is pointless to wait for Artificial Intelligence to become smarter than us; we must control it as it develops.
Hinton did not sign the letter subscribed by more than a thousand AI experts requesting a six-month moratorium on research. His reason is that he believes the approach is completely naive and there is no way it could be implemented. There are reasons he argues that are very important: even saving the competition between large companies, there is also that between countries. If the U.S. decided to stop developing Artificial Intelligence, do we really believe that China would stop? The idea of halting research brings attention to the problem, but it won’t happen. With nuclear weapons, since people realized we would all lose in a nuclear war, treaties were possible. With Artificial Intelligence, it will be much more complicated because it is very difficult to verify if people are working on it.
The best thing that can be recommended is for many very intelligent people to try to figure out how to contain the dangers of these things. Artificial Intelligence is a fantastic technology, it is causing great advances in medicine, the development of new materials, forecasting earthquakes or floods… We need a lot of work to understand how to contain AI. It is pointless to wait for AI to be smarter than us; we must control it as it develops. We also need to understand how to contain it, how to prevent its bad consequences. For example, I believe all governments should insist that all fake images have a label.
Finally, Hinton has pointed out: “We have the opportunity to prepare for this challenge. We need many creative and intelligent people. If there is any way to keep AI under control, we need to figure it out before it becomes too intelligent.”